MMACE Paper: Graph Neural Network for HIV Inhibition
Show code cell source
# import os
# os.environ["CUDA_VISIBLE_DEVICES"] = "2"
import pandas as pd
import matplotlib.pyplot as plt
import seaborn as sns
import matplotlib as mpl
import numpy as np
import tensorflow as tf
import selfies as sf
import exmol
import skunk
import warnings
from rdkit import Chem
from rdkit.Chem.Draw import rdDepictor
rdDepictor.SetPreferCoordGen(True)
from rdkit.Chem.Draw import IPythonConsole
IPythonConsole.ipython_useSVG = True
sns.set_context("notebook")
sns.set_style(
"dark",
{
"xtick.bottom": True,
"ytick.left": True,
"xtick.color": "#666666",
"ytick.color": "#666666",
"axes.edgecolor": "#666666",
"axes.linewidth": 0.8,
"figure.dpi": 300,
},
)
color_cycle = ["#1BBC9B", "#F06060", "#F3B562", "#6e5687", "#5C4B51"]
mpl.rcParams["axes.prop_cycle"] = mpl.cycler(color=color_cycle)
np.random.seed(0)
2024-11-22 06:53:49.666154: I external/local_xla/xla/tsl/cuda/cudart_stub.cc:32] Could not find cuda drivers on your machine, GPU will not be used.
2024-11-22 06:53:49.669420: I external/local_xla/xla/tsl/cuda/cudart_stub.cc:32] Could not find cuda drivers on your machine, GPU will not be used.
2024-11-22 06:53:49.676319: E external/local_xla/xla/stream_executor/cuda/cuda_fft.cc:477] Unable to register cuFFT factory: Attempting to register factory for plugin cuFFT when one has already been registered
WARNING: All log messages before absl::InitializeLog() is called are written to STDERR
E0000 00:00:1732258429.689897 1865 cuda_dnn.cc:8310] Unable to register cuDNN factory: Attempting to register factory for plugin cuDNN when one has already been registered
E0000 00:00:1732258429.694156 1865 cuda_blas.cc:1418] Unable to register cuBLAS factory: Attempting to register factory for plugin cuBLAS when one has already been registered
2024-11-22 06:53:49.710319: I tensorflow/core/platform/cpu_feature_guard.cc:210] This TensorFlow binary is optimized to use available CPU instructions in performance-critical operations.
To enable the following instructions: AVX2 FMA, in other operations, rebuild TensorFlow with the appropriate compiler flags.
# shuffle rows and sample fom HIV dataset
hivdata = pd.read_csv("HIV.csv")
# REDUCED Data FOR CI
hivdata = hivdata.sample(frac=0.1).reset_index(drop=True)
hivdata.head()
smiles | activity | HIV_active | |
---|---|---|---|
0 | COc1ccc2c(c1)OCC1(C)c3cc(O)c(OCc4ccccc4)cc3OC21 | CI | 0 |
1 | Cc1cc(-c2ccc(Cl)cc2)c(C#N)c(=S)n1C1OC(CO)C(O)C... | CI | 0 |
2 | CNn1c(-c2ccccc2)n[nH]c1=O | CM | 1 |
3 | O=NN(CCCl)C(=O)NC1CCCCC1 | CI | 0 |
4 | C=C1CCC(C)(c2ccc(CO)cc2)C1C | CI | 0 |
def gen_smiles2graph(sml):
"""Argument for the RD2NX function should be a valid SMILES sequence
returns: the graph
"""
m, smi_canon, status = exmol.stoned.sanitize_smiles(sml)
# m = Chem.MolFromSmiles(smi_canon)
m = Chem.AddHs(m)
order_string = {
Chem.rdchem.BondType.SINGLE: 1,
Chem.rdchem.BondType.DOUBLE: 2,
Chem.rdchem.BondType.TRIPLE: 3,
Chem.rdchem.BondType.AROMATIC: 4,
}
N = len(list(m.GetAtoms()))
# nodes = np.zeros((N,100))
nodes = np.zeros((440, 100))
for i in m.GetAtoms():
nodes[i.GetIdx(), i.GetAtomicNum()] = 1
# adj = np.zeros((N,N))
adj = np.zeros((440, 440))
for j in m.GetBonds():
u = min(j.GetBeginAtomIdx(), j.GetEndAtomIdx())
v = max(j.GetBeginAtomIdx(), j.GetEndAtomIdx())
order = j.GetBondType()
if order in order_string:
order = order_string[order]
else:
raise Warning("Ignoring bond order" + order)
adj[u, v] = 1
adj[v, u] = 1
adj += np.eye(440)
return nodes, adj
class GCNLayer(tf.keras.layers.Layer):
"""Implementation of GCN as layer"""
def __init__(self, activation=None, **kwargs):
# constructor, which just calls super constructor
# and turns requested activation into a callable function
super(GCNLayer, self).__init__(**kwargs)
self.activation = tf.keras.activations.get(activation)
def build(self, input_shape):
# create trainable weights
node_shape, adj_shape = input_shape
self.w = self.add_weight(shape=(node_shape[2], node_shape[2]), name="w")
def call(self, inputs):
# split input into nodes, adj
nodes, adj = inputs
# compute degree
degree = tf.reduce_sum(adj, axis=-1)
# GCN equation
new_nodes = tf.einsum("bi,bij,bjk,kl->bil", 1 / degree, adj, nodes, self.w)
out = self.activation(new_nodes)
return out, adj
class GRLayer(tf.keras.layers.Layer):
"""Reduction layer: A GNN layer that computes average over all node features"""
def __init__(self, name="GRLayer", **kwargs):
super(GRLayer, self).__init__(name=name, **kwargs)
def call(self, inputs):
nodes, adj = inputs
reduction = tf.reduce_mean(nodes, axis=1)
return reduction
ninput = tf.keras.Input(
(
None,
100,
)
)
ainput = tf.keras.Input(
(
None,
None,
)
)
# GCN block
x = GCNLayer("relu")([ninput, ainput])
x = GCNLayer("relu")(x)
x = GCNLayer("relu")(x)
x = GCNLayer("relu")(x)
# reduce to graph features
x = GRLayer()(x)
# standard layers
x = tf.keras.layers.Dense(256)(x)
x = tf.keras.layers.Dense(1, activation="sigmoid")(x)
gcnmodel = tf.keras.Model(inputs=(ninput, ainput), outputs=x)
gcnmodel.compile(
"adam",
loss=tf.keras.losses.BinaryCrossentropy(from_logits=False),
metrics=["accuracy"],
)
gcnmodel.summary()
2024-11-22 06:53:52.117248: E external/local_xla/xla/stream_executor/cuda/cuda_driver.cc:152] failed call to cuInit: INTERNAL: CUDA error: Failed call to cuInit: UNKNOWN ERROR (303)
Model: "functional"
┏━━━━━━━━━━━━━━━━━━━━━┳━━━━━━━━━━━━━━━━━━━┳━━━━━━━━━━━━┳━━━━━━━━━━━━━━━━━━━┓ ┃ Layer (type) ┃ Output Shape ┃ Param # ┃ Connected to ┃ ┡━━━━━━━━━━━━━━━━━━━━━╇━━━━━━━━━━━━━━━━━━━╇━━━━━━━━━━━━╇━━━━━━━━━━━━━━━━━━━┩ │ input_layer │ (None, None, 100) │ 0 │ - │ │ (InputLayer) │ │ │ │ ├─────────────────────┼───────────────────┼────────────┼───────────────────┤ │ input_layer_1 │ (None, None, │ 0 │ - │ │ (InputLayer) │ None) │ │ │ ├─────────────────────┼───────────────────┼────────────┼───────────────────┤ │ gcn_layer │ [(None, None, │ 10,000 │ input_layer[0][0… │ │ (GCNLayer) │ 100), (None, │ │ input_layer_1[0]… │ │ │ None, None)] │ │ │ ├─────────────────────┼───────────────────┼────────────┼───────────────────┤ │ gcn_layer_1 │ [(None, None, │ 10,000 │ gcn_layer[0][0], │ │ (GCNLayer) │ 100), (None, │ │ gcn_layer[0][1] │ │ │ None, None)] │ │ │ ├─────────────────────┼───────────────────┼────────────┼───────────────────┤ │ gcn_layer_2 │ [(None, None, │ 10,000 │ gcn_layer_1[0][0… │ │ (GCNLayer) │ 100), (None, │ │ gcn_layer_1[0][1] │ │ │ None, None)] │ │ │ ├─────────────────────┼───────────────────┼────────────┼───────────────────┤ │ gcn_layer_3 │ [(None, None, │ 10,000 │ gcn_layer_2[0][0… │ │ (GCNLayer) │ 100), (None, │ │ gcn_layer_2[0][1] │ │ │ None, None)] │ │ │ ├─────────────────────┼───────────────────┼────────────┼───────────────────┤ │ GRLayer (GRLayer) │ (None, 100) │ 0 │ gcn_layer_3[0][0… │ │ │ │ │ gcn_layer_3[0][1] │ ├─────────────────────┼───────────────────┼────────────┼───────────────────┤ │ dense (Dense) │ (None, 256) │ 25,856 │ GRLayer[0][0] │ ├─────────────────────┼───────────────────┼────────────┼───────────────────┤ │ dense_1 (Dense) │ (None, 1) │ 257 │ dense[0][0] │ └─────────────────────┴───────────────────┴────────────┴───────────────────┘
Total params: 66,113 (258.25 KB)
Trainable params: 66,113 (258.25 KB)
Non-trainable params: 0 (0.00 B)
def gen_data():
for i in range(len(hivdata)):
graph = gen_smiles2graph(hivdata.smiles[i])
activity = hivdata.HIV_active[i]
yield graph, activity
data = tf.data.Dataset.from_generator(
gen_data,
output_types=((tf.float32, tf.float32), tf.float32),
output_shapes=(
(tf.TensorShape([None, 100]), tf.TensorShape([None, None])),
tf.TensorShape([]),
),
)
N = len(hivdata)
split = int(0.1 * N)
test_data = data.take(split)
nontest = data.skip(split)
val_data, train_data = nontest.take(split), nontest.skip(split).shuffle(1000)
class_weight = {0: 1.0, 1: 30.0} # to account for class imbalance
result = gcnmodel.fit(
train_data.batch(128),
validation_data=val_data.batch(128),
epochs=30,
verbose=0,
class_weight=class_weight,
)
2024-11-22 06:54:11.690013: I tensorflow/core/framework/local_rendezvous.cc:405] Local rendezvous is aborting with status: OUT_OF_RANGE: End of sequence
[[{{node IteratorGetNext}}]]
/opt/hostedtoolcache/Python/3.11.10/x64/lib/python3.11/contextlib.py:158: UserWarning: Your input ran out of data; interrupting training. Make sure that your dataset or generator can generate at least `steps_per_epoch * epochs` batches. You may need to use the `.repeat()` function when building your dataset.
self.gen.throw(typ, value, traceback)
2024-11-22 06:54:13.555166: I tensorflow/core/framework/local_rendezvous.cc:405] Local rendezvous is aborting with status: OUT_OF_RANGE: End of sequence
[[{{node IteratorGetNext}}]]
2024-11-22 06:54:32.998708: I tensorflow/core/framework/local_rendezvous.cc:405] Local rendezvous is aborting with status: OUT_OF_RANGE: End of sequence
[[{{node IteratorGetNext}}]]
2024-11-22 06:55:12.569708: I tensorflow/core/framework/local_rendezvous.cc:405] Local rendezvous is aborting with status: OUT_OF_RANGE: End of sequence
[[{{node IteratorGetNext}}]]
2024-11-22 06:56:30.212414: I tensorflow/core/framework/local_rendezvous.cc:405] Local rendezvous is aborting with status: OUT_OF_RANGE: End of sequence
[[{{node IteratorGetNext}}]]
2024-11-22 06:59:07.961691: I tensorflow/core/framework/local_rendezvous.cc:405] Local rendezvous is aborting with status: OUT_OF_RANGE: End of sequence
[[{{node IteratorGetNext}}]]
fig, (ax1, ax2) = plt.subplots(1, 2, figsize=(8, 4))
ax1.plot(result.history["loss"], label="training")
ax1.plot(result.history["val_loss"], label="validation")
ax1.legend()
ax1.set_xlabel("Epoch")
ax1.set_ylabel("Loss")
ax2.plot(result.history["accuracy"], label="training")
ax2.plot(result.history["val_accuracy"], label="validation")
ax2.legend()
ax2.set_xlabel("Epoch")
ax2.set_ylabel("Accuracy")
fig.tight_layout()
fig.savefig("gnn-loss-acc.png", dpi=180)
fig.show()
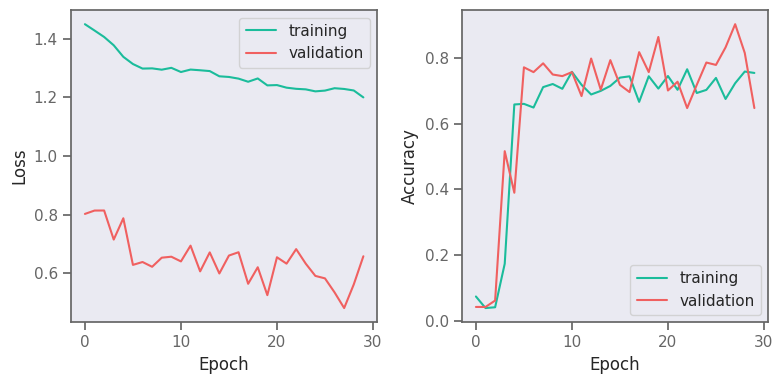
from sklearn.metrics import roc_curve
from sklearn.metrics import auc
prediction = []
test_y = []
for x, y in test_data.as_numpy_iterator():
yhat = gcnmodel((x[0][np.newaxis, ...], x[1][np.newaxis, ...]))
prediction.append(yhat.numpy())
test_y.append(y)
prediction = np.array(prediction).flatten()
test_y = np.array(test_y)
fpr_keras, tpr_keras, thresholds_keras = roc_curve(test_y, prediction)
auc_keras = auc(fpr_keras, tpr_keras)
plt.figure(figsize=(6, 4), dpi=100)
plt.plot(fpr_keras, tpr_keras, label="AUC = {:.3f}".format(auc_keras))
plt.plot([0, 1], [0, 1], linestyle="--")
plt.xlabel("True Positive Rate")
plt.ylabel("False Positive Rate")
plt.legend()
plt.savefig("gnn-roc.png", dpi=300)
plt.show()
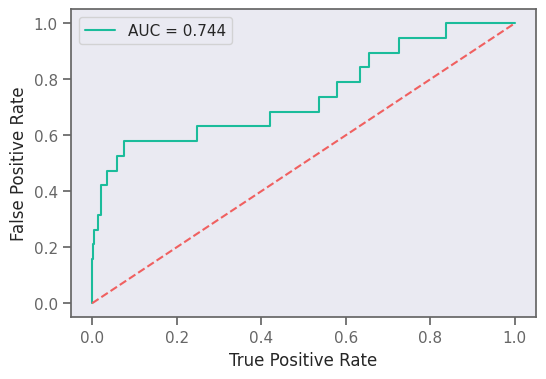
CF explanation
The following example find CFs for a given molecule where the HIV activity is zero.
def predictor_function(smiles, selfies):
# print('inut:',smiles)
labels = []
for sml in smiles:
nodes, adj_mat = gen_smiles2graph(sml)
pred = gcnmodel((nodes[np.newaxis, ...], adj_mat[np.newaxis, ...])).numpy()
labels.append(pred)
labels = np.array(labels).flatten()
bin_labels = np.where(labels > 0.5, np.ones(len(labels)), np.zeros(len(labels)))
target_act = np.zeros(len(labels))
return abs(bin_labels - target_act).astype(bool)
basic = exmol.get_basic_alphabet()
stoned_kwargs = {"num_samples": 1500, "alphabet": basic, "max_mutations": 2}
example_base = "C=CCN(CC=C)C(=O)Nc1ccc(C(=O)NN=Cc2cccc(OC)c2OC)cc1"
space = exmol.sample_space(
example_base,
predictor_function,
stoned_kwargs={"num_samples": 1500, "alphabet": basic, "max_mutations": 2},
quiet=True,
)
exps = exmol.cf_explain(space)
fkw = {"figsize": (8, 6)}
mpl.rc("axes", titlesize=12)
exmol.plot_cf(exps, figure_kwargs=fkw, mol_size=(450, 400), nrows=1)
plt.savefig("gnn-simple.png", dpi=180)
svg = exmol.insert_svg(exps, mol_fontsize=16)
with open("gnn-simple.svg", "w") as f:
f.write(svg)
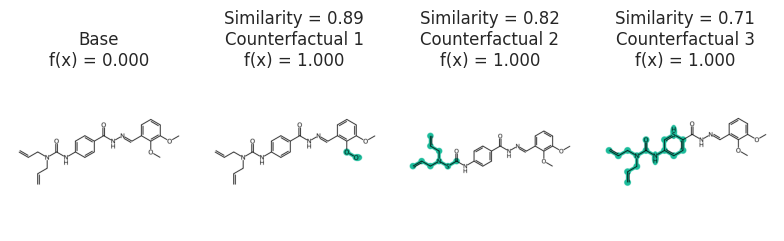
font = {"family": "normal", "weight": "normal", "size": 22}
exmol.plot_space(
space,
exps,
figure_kwargs=fkw,
mol_size=(300, 200),
offset=0,
cartoon=True,
rasterized=True,
)
plt.scatter([], [], label="Counterfactual", s=150, color=plt.get_cmap("viridis")(1.0))
plt.scatter([], [], label="Same Class", s=150, color=plt.get_cmap("viridis")(0.0))
plt.legend(fontsize=22)
plt.tight_layout()
svg = exmol.insert_svg(exps, mol_fontsize=16)
with open("gnn-space.svg", "w") as f:
f.write(svg)
/tmp/ipykernel_1865/1297770767.py:14: UserWarning: Tight layout not applied. The left and right margins cannot be made large enough to accommodate all Axes decorations.
plt.tight_layout()
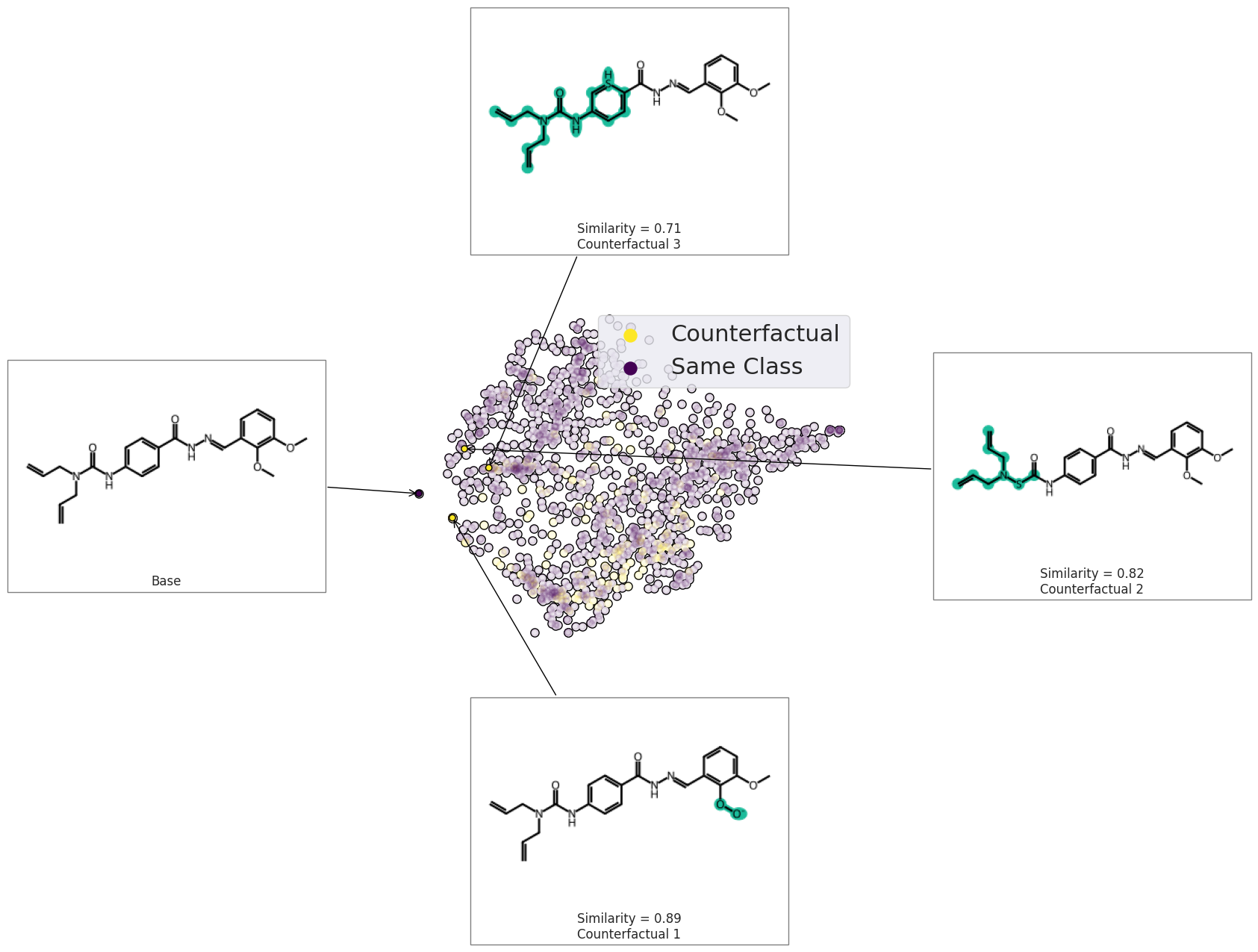
exps = exmol.cf_explain(space, nmols=19)
fkw = {"figsize": (12, 10)}
mpl.rc("axes", titlesize=10)
exmol.plot_cf(
exps, figure_kwargs=fkw, mol_size=(450, 400), mol_fontsize=26, nrows=4, ncols=5
)
plt.savefig("gnn-simple-20.png", bbox_inches="tight", dpi=300)
svg = exmol.insert_svg(exps, mol_fontsize=14)
with open("gnn-simple-20.svg", "w") as f:
f.write(svg)
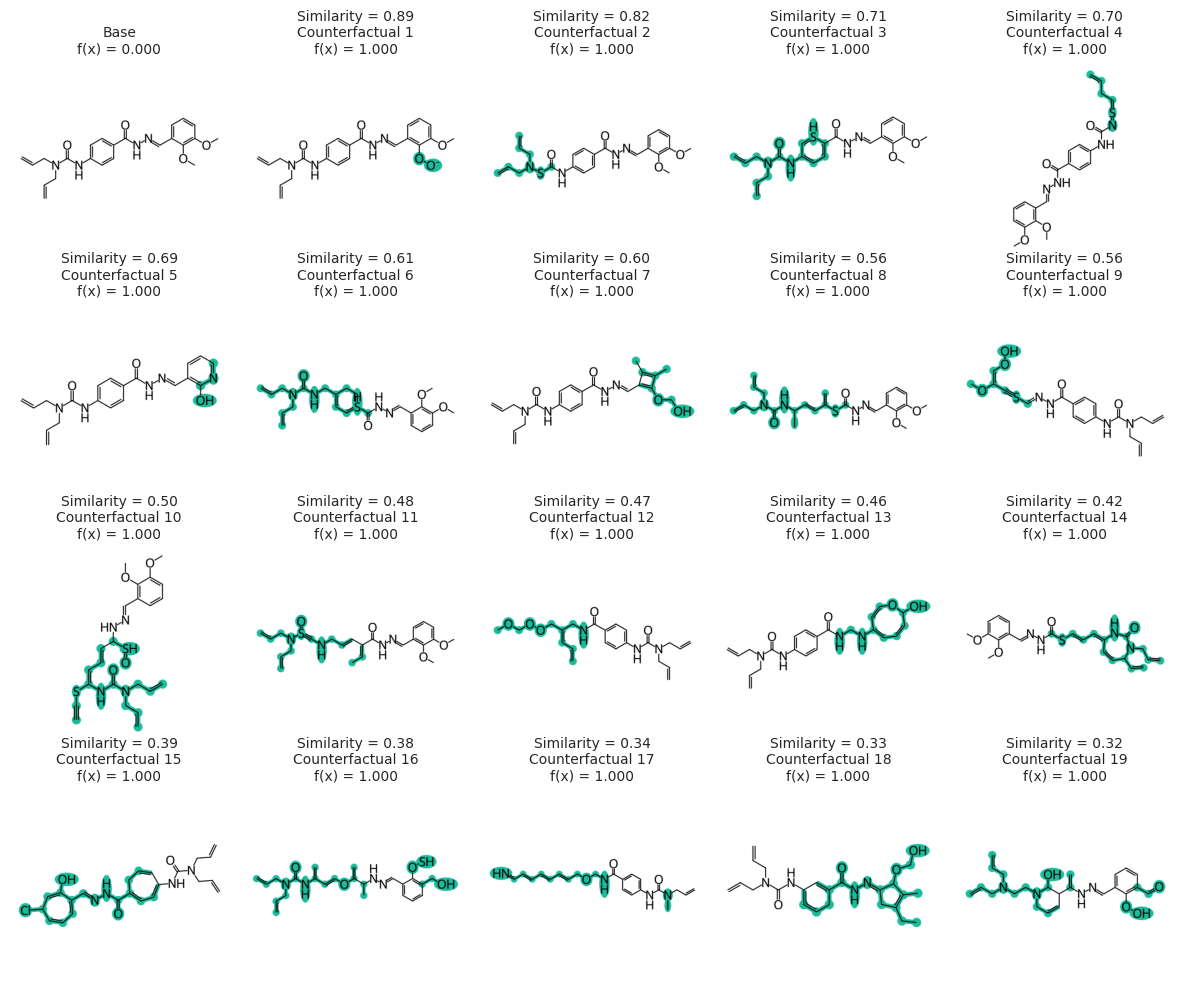
fkw = {"figsize": (8, 6)}
font = {"family": "normal", "weight": "normal", "size": 22}
exmol.plot_space(space, exps, figure_kwargs=fkw, mol_size=(350, 300), mol_fontsize=22)
plt.scatter([], [], label="Same Label", s=150, color=plt.get_cmap("viridis")(1.0))
plt.scatter([], [], label="Counterfactual", s=150, color=plt.get_cmap("viridis")(0.0))
plt.legend(fontsize=22)
plt.savefig("gnn-space.png", bbox_inches="tight", dpi=180)
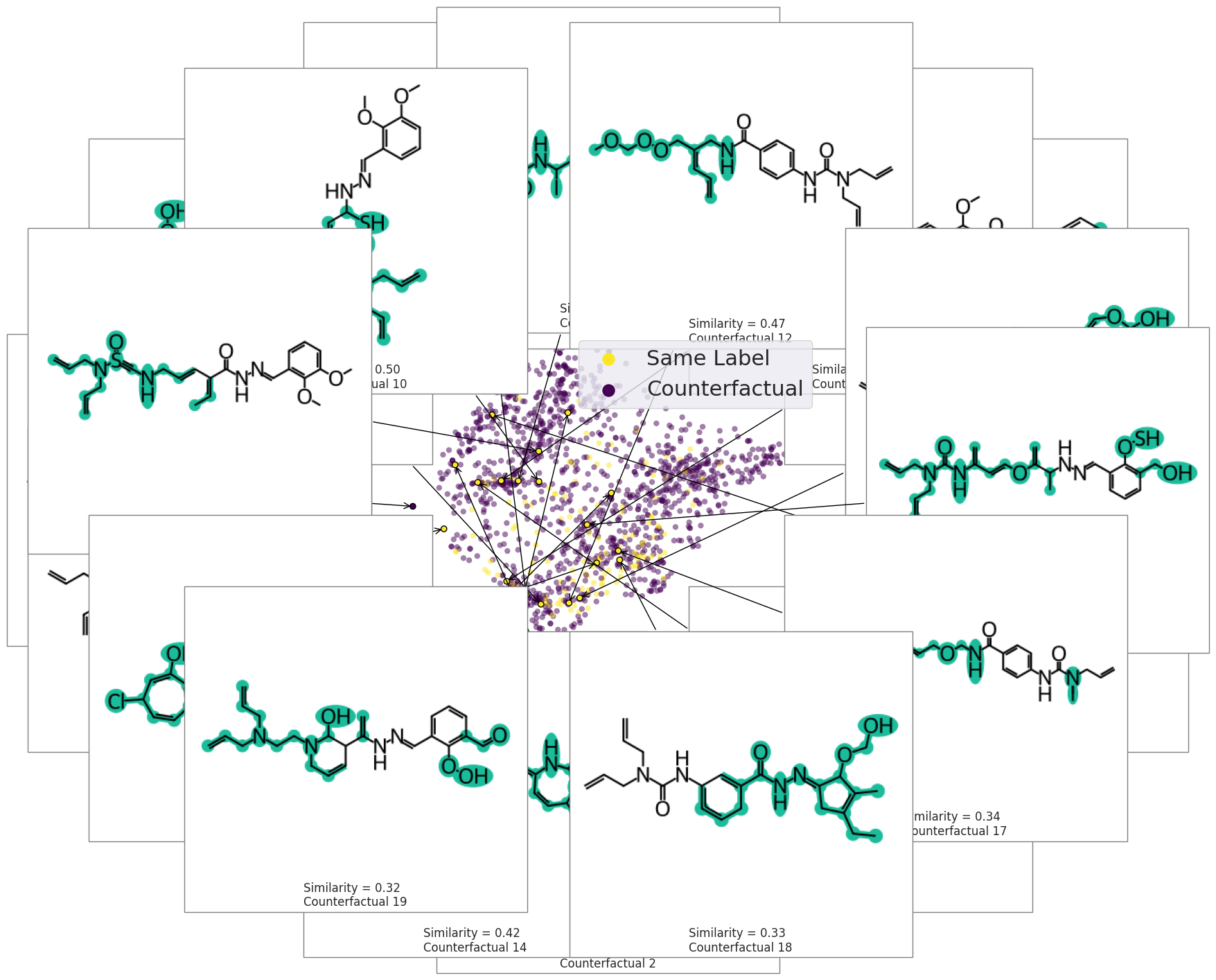