Tutorial
We’ll show here how to explain molecular property prediction tasks without access to the gradients or any properties of a molecule. To set-up this activity, we need a black box model. We’ll use something simple here – the model is classifier that says if a molecule has an alcohol (1) or not (0). Let’s implement this model first
from rdkit import Chem
from rdkit.Chem.Draw import IPythonConsole
# set-up rdkit drawing preferences
IPythonConsole.ipython_useSVG = True
IPythonConsole.drawOptions.drawMolsSameScale = False
def model(smiles):
mol = Chem.MolFromSmiles(smiles)
match = mol.GetSubstructMatches(Chem.MolFromSmarts("[O;!H0]"))
return 1 if match else 0
Let’s now try it out on some molecules
smi = "CCCCCCO"
print("f(s)", model(smi))
Chem.MolFromSmiles(smi)
f(s) 1
smi = "OCCCCCCO"
print("f(s)", model(smi))
Chem.MolFromSmiles(smi)
f(s) 1
smi = "c1ccccc1"
print("f(s)", model(smi))
Chem.MolFromSmiles(smi)
f(s) 0
Counterfacutal explanations
Let’s now explain the model - pretending we don’t know how it works - using counterfactuals
import exmol
instance = "CCCCCCO"
space = exmol.sample_space(instance, model, batched=False)
cfs = exmol.cf_explain(space, 1)
exmol.plot_cf(cfs)
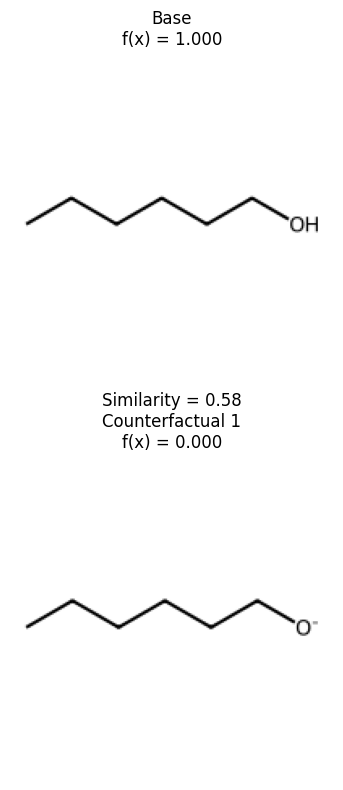
We can see that removing the alcohol is the smallest change to affect the prediction of this molecule. Let’s see the space and look at where these counterfactuals are.
exmol.plot_space(space, cfs)
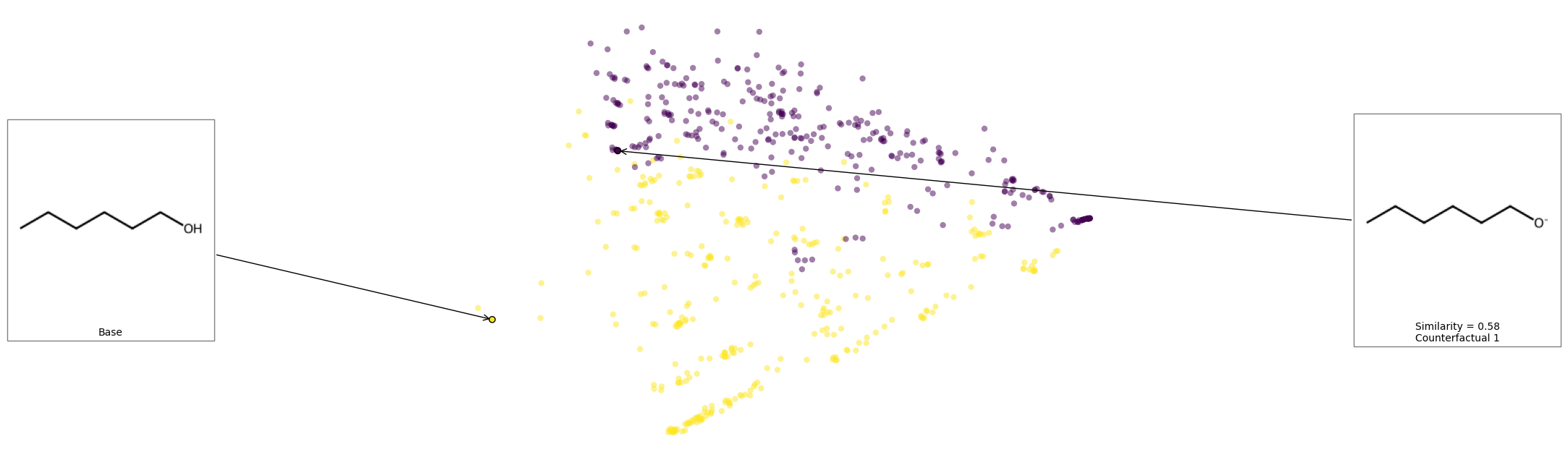
Explain using substructures
Now we’ll try to explain our model using substructures.
exmol.lime_explain(space)
exmol.plot_descriptors(space)
SMARTS annotations for MACCS descriptors were created using SMARTSviewer (smartsview.zbh.uni-hamburg.de, Copyright: ZBH, Center for Bioinformatics Hamburg) developed by K. Schomburg et. al. (J. Chem. Inf. Model. 2010, 50, 9, 1529–1535)
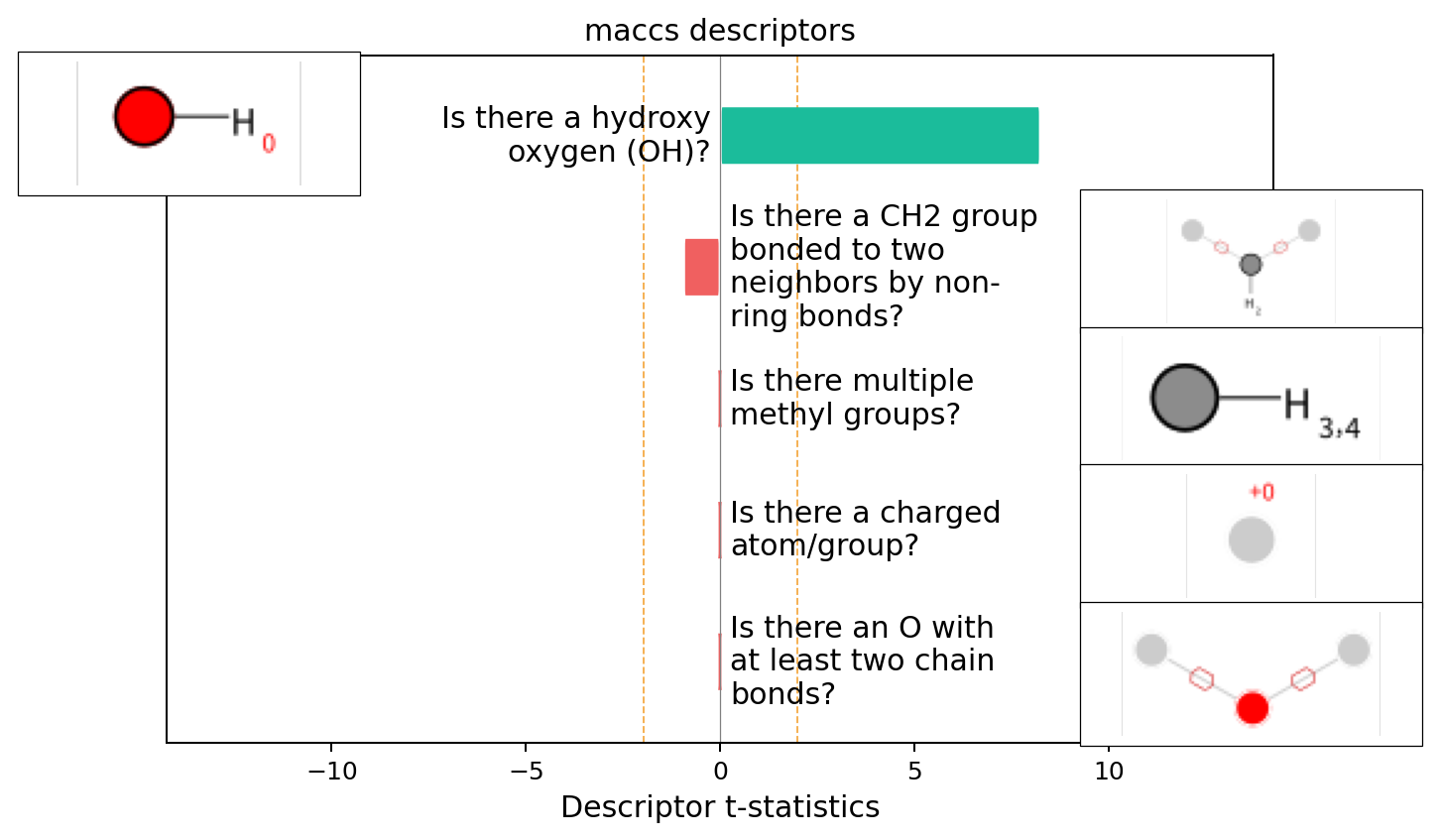
This seems like a pretty clear explanation. Let’s take a look at using substructures that are present in the molecule
import skunk
exmol.lime_explain(space, descriptor_type="ECFP")
svg = exmol.plot_descriptors(space, return_svg=True)
skunk.display(svg)
svg = exmol.plot_utils.similarity_map_using_tstats(space[0], return_svg=True)
skunk.display(svg)
We can see that most of the model is explained from the presence of the alcohol group - as expected.
Text
We can prepare a natural language summary of these results using exmol
:
exmol.lime_explain(space, descriptor_type="ECFP")
e = exmol.text_explain(space)
for ei in e:
print(ei[0], end="")
Is there primary alcohol? Yes and this is positively correlated with property. This is very important for the property
To prepare the natural language summary, we need to convert to a prompt that a model like GPT-3 can parse. Insert the output below into a language model to get a summary.
Or you can pass it directly, by installing the langchain
package and setting-up an openai key
print(exmol.text_explain_generate(e, property_name="active"))
The presence of a primary alcohol group in the molecule is crucial for its "active" property. This functional group is positively correlated with the activity, suggesting that it plays a significant role in the molecule's interaction with its target or environment. The hydroxyl group in the primary alcohol can participate in hydrogen bonding, enhancing the molecule's solubility and reactivity, which are often essential for biological activity. If the primary alcohol were absent, the molecule might lack the necessary polarity and hydrogen bonding capability, potentially reducing its effectiveness or altering its mechanism of action. Therefore, the primary alcohol is a key structural feature that underpins the molecule's active property.